Battery Energy Storage System Management for Improving Power Grid Reliability
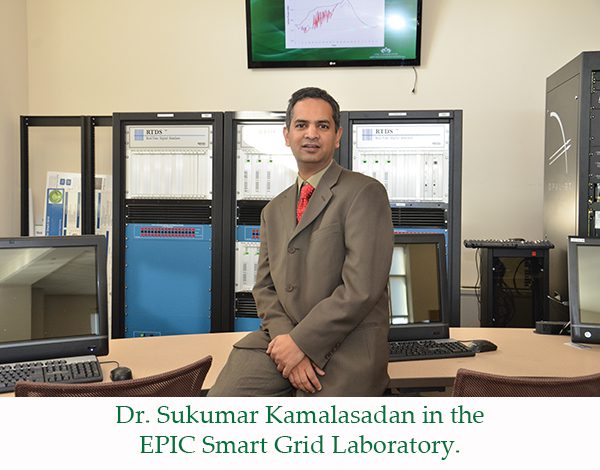
To improve the way solar power is integrated and distributed to the grid using grid level batteries, Electrical and Computer Engineering Associate Professor Dr. Sukumar Kamalasadan and his students have been performing research at Duke Energy’s photovoltaic and battery storage system testbed in Catawba County since 2013. The research has developed and quantified a number of cost-saving measures, and soon the value-management system will be integrated in the field for managing the solar power farm.
The Power Grid Connected Energy Storage Management project initially began as a battery storage system study for use with photovoltaics as part of grid modernization. The purpose was to solve the inconsistency problems of photovoltaics caused by weather changes. The researchers worked to balance solar power capacity by using batteries that are charged at times of excess solar output and then discharged when the power is needed on the grid.
“We decided to start at the Sherrills Ford distribution feeder, which has an existing PV farm with 1 MW installed capacity,” Dr. Kamalasadan said. “Nearby is a 250 KW/750Kwh battery storage facility. Our EPIC (Energy Production and Infrastructure Center) researchers had expertise in renewables, and we formed a team to develop an algorithm to smooth the output from solar energy. We concentrated on keeping voltage levels consistent when the PV output changes due to weather and simultaneously used the battery power to feed the feeder load during peak-load times.”
The first step in the research was to gather field data to be used in building the feeder model and control algorithm. Based on the data, the team developed a control strategy for more evenly distributing the photovoltaic output.
“First, in 2013-14, we developed a system to transfer the data through the internet to the Smart Grid lab at UNC Charlotte. After the communication link was successfully established we designed the initial testbed and evaluated the proof-of-concept,” Dr. Kamalasadan said. “We then deployed the algorithm on a hardware controller to connect to the battery in the field. The results showed a much more efficient control performance than sending a signal from the UNCC campus.”
Then the team developed a stacked algorithm to optimize battery charge and simultaneously supply battery power with three goals in mind, to smooth the PV farm output, regulate the voltage, and feed the feeder load at the peak power usage time. In 2015, they integrated historical weather patterns into the algorithm to optimize the amount of charge the batteries had at the start of the day, so that they would have the power needed for distribution on cloudy days or the capacity needed for storage on sunny days.
“This was very challenging,” Dr. Kamalasadan said. “There are always more variables to control in real life than what you plan for in the lab.”
In 2016, the team looked at the actual dollar value of using battery storage with the stacked algorithm to smooth PV output, and at regulating the voltage and sending power from the battery during peak load. Five main power quality and energy management areas for monetary savings were identified and savings were calculated. The team also looked at how well the controllers performed in maintaining the voltage interacting with other controllers in the field.
The next step in the project will be to develop a fully integrated value management system for use in the distribution system that will both manage the photovoltaics and calculate the overall commercial value.
“Overall Duke Energy has benefitted in optimizing the efficiency of the feeder when the PV farms are integrated,” Dr. Kamalasadan said, “and UNC Charlotte has benefitted in being able to provide this wonderful learning experience to our students. What I like about this project more than anything else is that we converted the algorithm into a value-based approach that could quantify the actual savings. We demonstrated a strong business case model that shows how the algorithm can be deployed in the field based on monetary value.”